Tools Thoughtworks Technology Radar 48. Conftest Trial Conftest is a tool for writing tests against structured configuration data. It relies on the Rego language from Open Policy Agent to write tests for Kubernetes configurations, Tekton pipeline definitions or even Terraform plans. We’ve had great experiences with Conftest — and its shallow learning curve. With fast feedback from tests, our teams iterate quickly and safely on configuration changes to Kubernetes. 49. kube-score Trial kube-score is a tool that does static code analysis of your Kubernetes object definitions. The output is a list of recommendations for what you can improve to make your application more secure and resilient. It has a list of predefined checks which includes best practices such as running containers with non-root privileges and correctly specifying resource limits. It’s been around for some time, and we’ve used it in a few projects as part of a CD pipeline for Kubernetes manifests. A major drawback of kube-score is that you can’t add custom policies. We typically supplement it with tools like Conftest in these cases. 50. Lighthouse Trial Lighthouse is a tool written by Google to assess web applications and web pages, collecting performance metrics and insights on good development practices. We’ve long advocated for performance testing as a first-class citizen, and the additions to Lighthouse that we mentioned five years ago certainly helped with that. Our thinking around architectural fitness functions created strong motivation for tools such as Lighthouse to be run in build pipelines. With the introduction of Lighthouse CI, it has become easier than ever to include Lighthouse in pipelines managed by various tools. 51. Metaflow Trial Metaflow is a user-friendly Python library and back-end service that helps data scientists and engineers build and manage production-ready data processing, ML training and inference workflows. Metaflow provides Python APIs that structure the code as a directed graph of steps. Each step can be decorated with flexible configurations such as the required compute and storage resources. Code and data artifacts for each step’s run (aka task) are stored and can be retrieved either for future runs or the next steps in the flow, enabling you to recover from errors, repeat runs and track versions of models and their dependencies across multiple runs. The value proposition of Metaflow is the simplicity of its idiomatic Python library: it fully integrates with the build and run-time infrastructure to enable running data engineering and science tasks in local and scaled production environments. At the time of writing, Metaflow is heavily integrated with AWS services such as S3 for its data store service and step functions for orchestration. Metaflow supports R in addition to Python. Its core features are open sourced. If you’re building and deploying your production ML and data-processing pipelines on AWS, Metaflow is a lightweight full-stack alternative framework to more complex platforms such as MLflow. © Thoughtworks, Inc. All Rights Reserved. 27
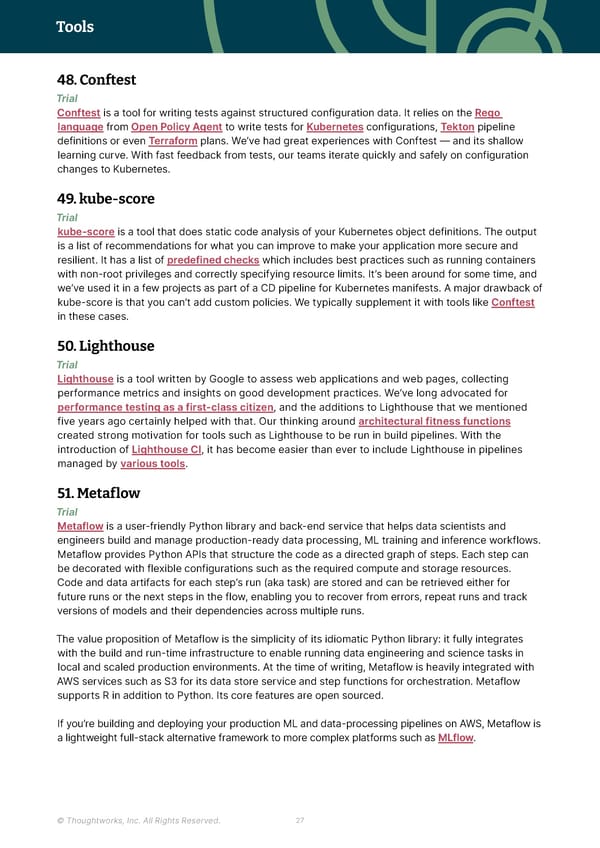