Themes AI-assisted software development To no one’s surprise, AI-related topics dominated our conversation for this edition of the Radar. For the first time ever, we needed a visual guide to untangle the different categories and capabilities (something we never had to resort to even in the heyday of chaos in the JavaScript ecosystem). As a software consultancy with a history of pioneering engineering practices like CI and CD, one of the categories of particular interest to us is using AI to assist in software development. As part of the Radar, we therefore discussed many coding assistance tools, like GitHub Copilot, Tabnine and Codeium. We’re also excited about how open-source LLMs for coding might shake up the tooling landscape, and we see great promise in the explosion of tools and capabilities for assistance beyond coding as well, such as user story writeup assistance, user research, elevator pitches and other language-based chores. At the same time, we hope developers use all of these tools responsibly and stay firmly in the driver’s seat, with things like hallucinated dependencies being just one of the security and quality risks to be aware of. How productive is measuring productivity? Software development can sometimes seem like magic to non-technologists, which leads managers to strive to measure just how productive developers are at their mysterious tasks. Our chief scientist, Martin Fowler, wrote about this topic as long ago as 2003, but it hasn’t gone away. We discussed many modern tools and techniques for this Radar that take more nuanced approaches to measuring the creative process of building software yet still remain inadequate. Fortunately, the industry has moved away from using lines of code as a measure of output. However, alternative ways to measure the A (“Activity”) of the SPACE framework, such as number of pull requests or issues resolved, are still poor indicators of productivity. Instead, the industry has started focusing on engineering effectiveness: rather than measure productivity, we should measure things we know contribute to or detract from the flow. Instead of focusing on an individual’s activities, we should focus on the sources of waste in the system and the conditions we can empirically show have an impact on the developer’s perception of “productivity.” New tools such as DX DevEx 360 address this by focusing on the developer experience rather than some specious measure of output. However, many leaders continue to refer to developer “productivity” in a vague, qualitative way. We suspect that at least some of this resurgence of interest concerns the impact of AI-assisted software development, which raises the inevitable question: is it having a positive impact? While measurements may be gaining some nuance, real measurements of productivity are still elusive. © Thoughtworks, Inc. All Rights Reserved. 6
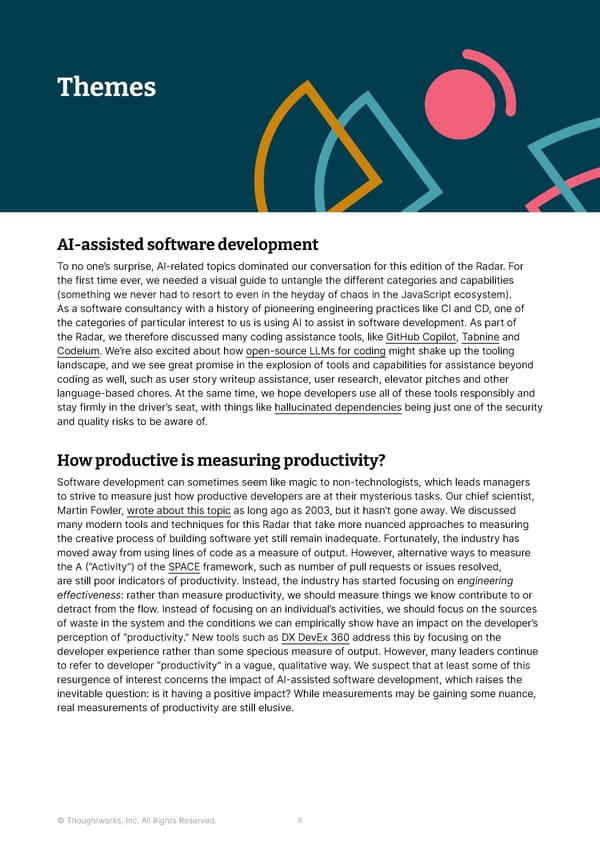